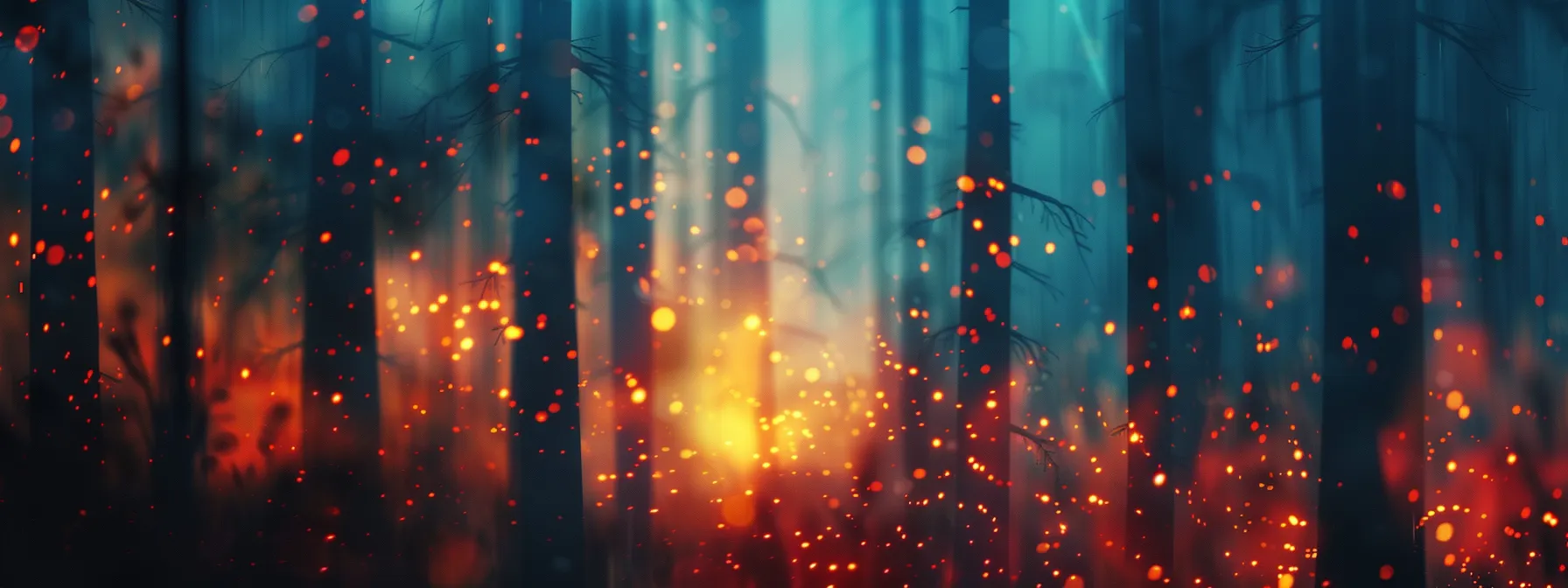
Insights Into Computer Vision’s Role in Fire Detection Innovations
Insights Into Computer Vision’s Role in Fire Detection Innovations
Innovations in Fire Detection: Computer Vision Insights
Computer vision radically improves fire detection systems. The article covers how advanced imaging transforms fire surveillance technology and reviews successful case studies that highlight real-world benefits. Readers will learn ways to overcome current challenges in fire detection and explore how technology meets regulatory standards. This clear overview addresses common safety concerns and offers practical solutions to boost system accuracy and efficiency.
Key Takeaways
- machine learning improves fire detection accuracy with advanced data sampling techniques
- automated systems integrate sensor data and aerial images for real-time hazard monitoring
- cloud-based methods ensure secure, continuous data validation and system calibration
- innovative detection models reduce false positives and negatives in surveillance
- collaboration between tech and fire services boosts operational readiness and safety compliance
Understand How Computer Vision Transforms Fire Detection Systems
Investigation covers how automation and advanced image processing techniques support fire detection systems. The analysis focuses on machine learning research, addressing overfitting and refining detection models with accurate data. This insight extends to real-time fire hazard analysis, enhanced by wavelet-based image techniques, ensuring expert knowledge in developing
.
Investigate the Role of Machine Learning in Fire Detection
Machine learning algorithms refine fire detection methods by processing sampling data from wireless sensor network devices and unmanned aerial vehicles, while guiding actuator responses to potential hazards. This approach shapes the detection process to ensure accuracy in identifying fire risks and supports real-time analytic outcomes through computer vision integration, leading to advanced monitoring solutions:
- Integration of unmanned aerial vehicle data for aerial monitoring
- Utilization of wireless sensor network inputs to verify fire incidents
- Control of actuator mechanisms to initiate prompt responses
- Application of precise sampling techniques for data validation
- Adjustment of system parameters to shape detection reliability
Assess Image Processing Techniques Used in Detection
Digital Fractal’s assessment of image processing techniques reveals a careful calibration of algorithms that meet regulation standards using a cloud-based approach, ensuring that each manuscript of data is validated through a secure cache and effective vehicle data integration:
- Adoption of precise sampling methods for accurate imaging
- Implementation of robust data cache mechanisms
- Use of cloud infrastructure for continuous monitoring
- Incorporation of vehicle-mounted sensors for live feedback
- Strict adherence to regulation requirements during deployment
Examine Real-Time Analysis of Fire Hazards
Digital Fractal’s approach to real-time analysis of fire hazards combines image processing with a search algorithm that optimizes precision and recall while managing system complexity, using techniques inspired by vggnet to refine detection accuracy. The process streamlines data captured from sensors and aerial units to support rapid hazard identification and response; consider the following steps to understand the workflow:
- Collect sensor data and integrate aerial monitoring inputs
- Apply a search algorithm to organize and analyze the data
- Utilize vggnet principles for enhanced image processing
- Adjust precision and recall settings for optimal detection
- Monitor system output to validate detection effectiveness
Highlight the Importance of Data Quality in Detection Models
Accurate measurement of data quality is critical for detection models, as adherence to institute of electrical and electronics engineers standards guarantees a reliable stream and sequence of inputs across diverse applications such as forestry and urban safety; this rigorous data handling process guides system calibration to meet specific detection needs:
Factor | Description |
---|---|
Institute of Electrical and Electronics Engineers | Sets standardized procedures for data validation |
Stream Data | Continuous input that supports real-time analysis |
Forestry | Application area demonstrating environmental vigilance |
Measurement | Quantifying the precision of data inputs for robust model training |
Sequence | Organizing data in a logical order to enhance model predictability |
Explore Key Innovations in Fire Surveillance Technology
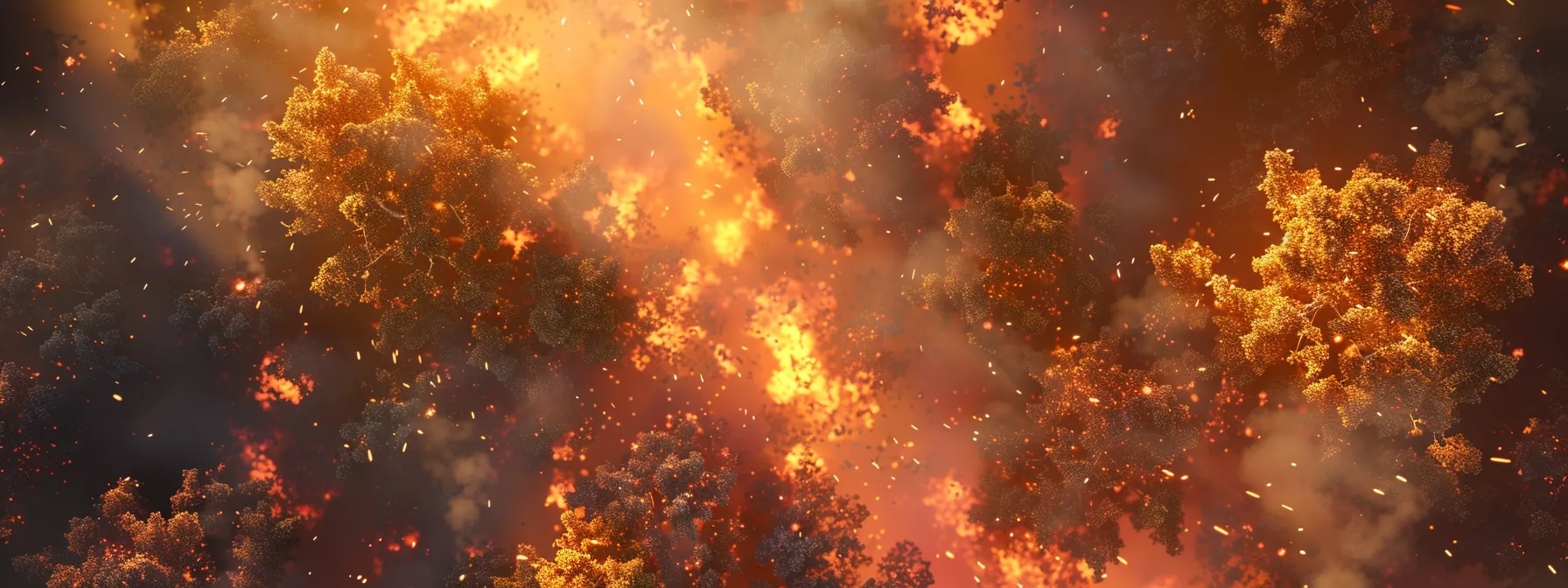
The section reviews innovations in fire surveillance, starting with advances in thermal imaging for fire prevention. It examines smart cameras using neural network principles like alexnet, drones that improve hazard monitoring, and IoT integration, backed by citation data to assess detection ratio. This overview sets the stage for detailed insights in each area.
Analyze Advances in Thermal Imaging for Fire Prevention
Digital Fractal evaluates how modern editing techniques combined with data from moderate resolution imaging spectroradiometer systems and advanced digital image processing sharpen the detection process, successfully reducing false positives and false negatives in fire surveillance. This approach refines thermal imaging to accurately identify a tree’s heat signature among other elements, offering practical benefits for users seeking reliable fire prevention measures.
Review Smart Cameras Designed for Fire Safety
Smart cameras engineered by Digital Fractal offer superior fire safety surveillance by integrating computer vision with javascript-based interfaces, ensuring accurate terrain analysis and strict adherence to ethics while also providing detailed paper documentation for review and certification:
- Seamless integration into existing surveillance networks
- Efficient javascript interfaces for real-time processing
- Accurate terrain assessment to detect fire hazards
- Comprehensive paper documentation for audit purposes
- Ethics-centric design that aligns with industry regulations
Evaluate Drones and Their Impact on Monitoring Fires
Digital Fractal’s evaluation of drones demonstrates significant advancements in fire monitoring by integrating aerial imaging to assess forest conditions, climate factors, and reproduction patterns; a robust literature review with insights from Springer Nature underpins their strategic effectiveness in real-time fire detection and response protocols:
Aspect | Description |
---|---|
Forest Analysis | Aerial imaging assists in identifying forest conditions critical to fire hazard assessment. |
Climate Impact | Integrates climate data to refine predictive models for fire outbreaks. |
Reproduction Patterns | Assesses vegetation reproduction to forecast and mitigate potential risks. |
Discuss Integration of IoT With Fire Detection Systems
Digital Fractal integrates IoT technology with fire detection systems to deliver accurate, real-time monitoring and an intuitive interface for analyzing smoke patterns. The approach combines open access sensor data with refined qin model calibration and softmax function parameters, ensuring detection methods are both responsive and reliable. This technique helps users verify potential risks with confidence and supports timely intervention:
Component | Description |
---|---|
Sensor Network | Gathers open access data for precise smoke and heat detection |
Qin Model Adjustments | Employs softmax function techniques to enhance data accuracy |
Interface Controls | Offers streamlined access to insights for prompt alert management |
Assess Challenges Facing Computer Vision in Fire Detection
The discussion highlights limitations in current technologies, reviews environmental impacts like rotation shifts during a forest fire, and considers data privacy concerns tied to image processing. The analysis references creative commons resources and insights from Muhammad, setting the structure for evaluating field use, external conditions, and privacy matters.
Identify Limitations of Current Technologies in Field Use
Current technologies in field use often face difficulties processing extensive sensor data, where limited backpropagation learning reduces the ability to extract precise fire information and compromises system adaptability, leaving practitioners seeking methods to overcome these obstacles:
Challenge | Explanation |
---|---|
Data Processing | Insufficient backpropagation learning limits the effective utilization of sensor information for fire detection |
Sensor Reliability | Variability and inaccuracies in sensor outputs hinder the accurate identification of fire events |
Information Flow | Restricted channels for processing and analyzing data slow down the overall system response during high-risk situations |
Review Environmental Factors Affecting Detection Accuracy
Digital Fractal’s analysis shows that environmental factors such as changing terrain behavior and light interference can distort detection signals, requiring adjustments in ensemble learning methods to refine accuracy. Studies referenced from pubmed and research by liu provide actionable insights that help optimize detection models, ensuring reliable operation even under challenging conditions.
Consider Data Privacy Issues Related to Image Processing
Digital Fractal acknowledges that data processing systems and intelligence applications, including transformer models and receptive field techniques, must address privacy concerns to safeguard sensitive image information while observing biodiversity trends. This careful approach ensures that image processing remains secure and compliant with industry standards:
- Robust data processing protocols
- Integration of advanced intelligence algorithms
- Optimization of receptive field measurements
- Implementation of transformer-based security measures
Learn About Successful Case Studies in Fire Detection
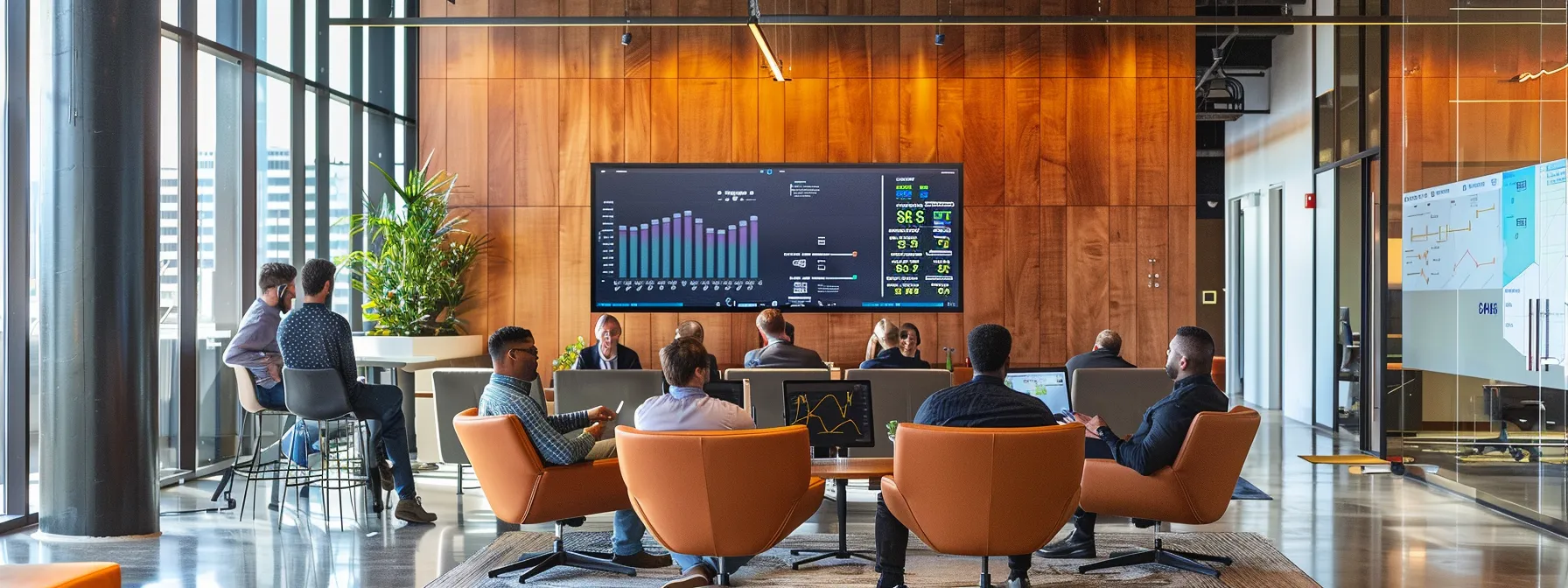
This section highlights case studies featuring
in urban areas, achievements in industrial fire safety solutions, and government initiatives promoting innovative detection. Topics include batch normalization, pattern recognition, loss function, dimension, and insights from nasa, demonstrating practical expertise in fire detection innovation.
Examine Implementation of Computer Vision in Urban Areas
Digital Fractal’s implementation of
in urban fire detection integrates machine learning with forecasting and heat analysis to provide decision-makers with actionable insights. A detailed pdf report synthesizes technical language and practical examples, showcasing how data from city sensors improves detection precision and system responsiveness.
Highlight Achievements in Industrial Fire Safety Solutions
Digital Fractal demonstrates expertise in industrial fire safety solutions by integrating advanced computer engineering and software systems that optimize environmental monitoring and response capabilities. Their approach employs precise sensor measurements to track humidity levels and calculate standard deviation for setting accurate alert thresholds, ensuring reliable protection that supports tourism infrastructure and critical industrial operations:
- Comprehensive sensor integration
- Real-time data analysis
- Efficient alert mechanisms
- Enhanced system reliability
- Optimized safety standards
Discuss Government Initiatives Promoting Innovative Detection
Government initiatives have advanced innovative detection by integrating aerial photography with deep learning models and computer science principles to develop a precise formula for fire detection. These efforts provide valuable attention to the needs of urban and industrial safety by offering real-time insights and practical solutions that improve system responsiveness and reliability.
Grasp the Future of Fire Detection Technology Trends
This section outlines the role of
in predictions, analyzes emerging technologies on the horizon, and discusses future collaboration between tech companies and fire services. Topics include optimizing design with ecology insights, improving fog and ycbcr imaging, and incorporating robot applications in fire detection systems.
Explore the Role of Artificial Intelligence in Predictions
Digital Fractal applies artificial intelligence to improve prediction accuracy in fire detection by using a tensorflow-based system that processes satellite data and provides timely evaluation and feedback, ensuring that memory for historical patterns optimizes current analysis:
Component | Description |
---|---|
Evaluation | Assesses sensor and computational performance in real time |
Feedback | Delivers immediate updates for system adjustments |
Satellite | Provides essential remote monitoring data |
TensorFlow | Drives machine learning algorithms for accurate predictions |
Memory | Stores historical trends to improve ongoing analysis |
Analyze Emerging Technologies on the Horizon
Emerging technologies drive improvements in fire detection by using refined computer vision insights that build on a robust data set and innovative methodology. These advancements, incorporating logistic regression models to process variations in soil conditions, support firefighter operations with actionable, real-time information and address industry needs effectively:
- Advanced data set integration for accurate monitoring
- Innovative methodology for enhanced detection precision
- Utilization of logistic regression to assess risk factors
- Monitoring soil parameters to identify potential hazards
- Support for firefighter safety through real-time insights
Discuss Future Collaboration Between Tech Companies and Fire Services
Digital Fractal foresees future collaboration between tech companies and fire services leading to innovations that reduce latency and enhance correlation between sensor inputs and response systems, thus sharpening perception for timely interventions. This partnership will prioritize sustainability using advanced vision transformer models to deliver precise, real-time fire risk assessments and improved operational readiness:
Aspect | Description |
---|---|
Latency | Optimizing system response time for immediate hazard recognition |
Correlation | Integrating data streams to validate fire risk detection efficiently |
Vision Transformer | Utilizing advanced models to enhance accuracy and perception in fire detection |
Understand Regulatory Standards Impacting Fire Detection Innovations
The analysis assesses current codes in fire safety technology adoption, reviews compliance challenges for new technologies, and explores future regulations shaping fire detection innovations. The content highlights significant factors such as activation function, basel, risk, function, and hyperparameter optimization, providing practical insight into evolving regulatory standards.
Assess Current Codes in Fire Safety Technology Adoption
Digital Fractal assesses current codes in fire safety technology adoption by consulting reliable literature and employing clear diagrams to illustrate emergency protocols and xml data interfaces for transport systems. The analysis guides stakeholders by presenting practical examples that simplify regulatory challenges and assist in aligning fire detection innovations with established standards:
- Thorough review of industry literature
- Use of detailed diagrams to depict emergency responses
- Incorporation of xml for seamless data transport
- Actionable insights for regulatory compliance
Review Compliance Challenges for New Technologies
Digital Fractal reviews compliance challenges for new technologies by maintaining strict policy adherence and utilizing predictive analytics to verify accurate motion detection data from each cell in the system. This method supports practical decision-making and efficient allocation of resource management while addressing potential regulatory hurdles. The approach is rooted in real-world application insights, ensuring fire detection innovations meet established guidelines without compromising system performance.
Explore Future Regulations Shaping Fire Detection Innovations
Digital Fractal investigates emerging regulatory standards that will define the future of fire detection innovations, emphasizing the integration of backbone network technologies and advanced machine vision that enhance property safety. The company underscores that rigorous computer vision protocols and classification techniques are essential for developing systems that not only comply with new regulations but also offer increased reliability in high-risk environments. This approach provides stakeholders with practical insights on how to design systems that balance technical advancement with compliance and operational efficiency.
Frequently Asked Questions
How does computer vision transform fire detection systems?
Computer vision transforms fire detection systems by enabling real-time monitoring and rapid identification of fire signatures, leading to faster alerts and more secure automated responses.
What new techniques boost fire surveillance technology?
Advanced sensors integrated with machine learning, real-time thermal imaging, and data-driven analytics offer swift incident response and accurate alerts.
What challenges affect computer vision in fire detection?
Computer vision for fire detection encounters difficulties such as variable lighting, smoke obscuring details, rapidly changing fire conditions, and the demand for swift, accurate analysis of limited, diverse imagery to ensure reliable results.
Which case studies display successful fire detection methods?
Recent case studies reveal that smart sensor integration in web applications, coupled with agile backend systems, provides rapid fire detection. These studies show that real-time monitoring and automated alerts lead to efficient response and mitigation.
What regulatory standards influence fire detection advancements?
Regulatory standards such as NFPA and UL shape fire detection advancements by establishing requirements for safety, functionality, and system performance, ensuring that innovative fire protection technologies meet verified benchmarks.
Conclusion
Innovations in fire detection using computer vision deliver faster and more precise hazard identification. The integration of advanced image processing, machine learning, and real-time sensor inputs fuels improvements in system reliability and performance. The use of smart cameras, drones, and IoT technologies further sharpens fire monitoring capabilities and supports effective response mechanisms. Stakeholders benefit from a comprehensive approach that couples technical innovation with regulatory compliance, ensuring valuable, actionable insights for enhanced safety. Digital Fractal Technologies is Ai consulting firm with experience in computer vision applications. We are based in Edmonton, Alberta, Canada. Contact us for your next project.