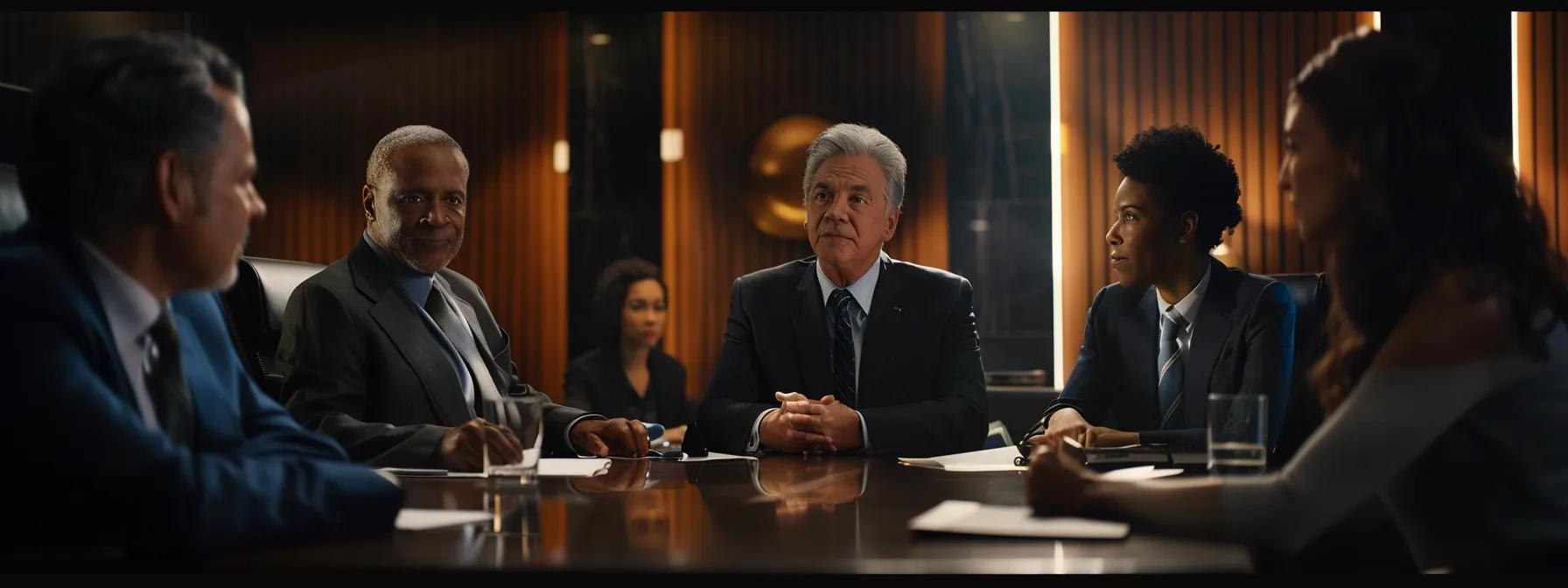
Exploring the Main Aspects of AI Consulting Services
By, digitalfractal
- 21 Jan, 2025
- 14 Views
- 0 Comment
In a rapidly changing business environment, many organizations struggle to harness the full potential of Artificial Intelligence (AI) to enhance operational efficiency and decision-making. This blog post will outline the typical scope of the AI consultancy process, from AI strategy development to pilot testing and implementation support. By understanding these stages, readers will gain insights into how to improve service delivery and effectively integrate AI solutions tailored to their unique needs. This content addresses common challenges in adopting AI, providing practical guidance for a successful transformation.
AI Strategy Developement
AI strategy development involves identifying relevant AI use cases across various departments, conducting a thorough needs assessment, and pinpointing processes where AI can deliver significant value. This approach ensures that organizations can develop tailored AI strategies and solutions that foster innovation and meet project requirements, such as those outlined in an RFP. For more detailed insights, consider exploring AI consulting services.
Identifying AI Use Cases in Various Departments
Identifying AI use cases in various departments is a crucial step in developing a successful AI strategy. Organizations should assess their specific needs, focusing on how AI frameworks can optimize operations and drive efficiency. By creating a comprehensive implementation roadmap, they can ensure that ethical AI principles, robust data strategies, and effective data governance are integrated, aligning projects with organizational goals and maximizing value across all sectors.
Department | AI Use Case | Expected Benefits |
---|---|---|
Marketing | Customer segmentation using predictive analytics | Improved targeting and engagement |
Human Resources | Automated resume screening | Faster hiring process |
Finance | Fraud detection algorithms | Reduced financial losses |
Operations | Predictive maintenance for equipment | Decreased downtime and costs |
Conducting Needs Assessment
Conducting a needs assessment is essential for developing a comprehensive AI strategy. This process involves analyzing current operations and identifying specific areas where AI can enhance efficiency while ensuring compliance with data privacy and data security regulations. A well-executed feasibility study helps organizations understand the implications of implementing AI, especially in sectors such as the public sector, where compliance is critical. By establishing a clear AI roadmap, they can strategically align AI initiatives with their business objectives, maximizing benefits and addressing potential challenges effectively.
Identifying Processes Where AI will Add Value
Identifying processes where AI can add value is essential for successful AI strategy development. Organizations often begin by assessing key areas that may benefit from machine learning applications, focusing on aspects such as pilot testing new solutions and implementing staff training programs to facilitate AI adoption. This proactive approach not only fosters capacity building within teams but also ensures that the integration of AI technologies aligns with overall business objectives, ultimately enhancing operational efficiency and effectiveness.
Feasibility Study for AI Projects
The feasibility study for AI projects involves identifying relevant AI use cases, such as natural language processing and computer vision, while analyzing their feasibility within the organization’s structure. It documents compliance requirements and conducts a risk and benefit analysis for each use case. Furthermore, it reviews the organization’s data strategy, data quality, and governance readiness for AI deployment, allowing for informed decisions based on impact and cost analysis.
Identify AI Use cases, analyzing feasibility
Identifying AI use cases and analyzing their feasibility is a crucial component of the feasibility study for AI projects. This process requires organizations to evaluate potential projects within the framework of project management principles, ensuring alignment with GDPR and data protection regulations. By incorporating a pilot implementation phase, teams can assess the practicality and effectiveness of AI solutions before a full-scale rollout, facilitating knowledge transfer and minimizing implementation risks.
Document compliance requirements
Documenting compliance requirements is a fundamental aspect of the feasibility study for AI projects. This process involves outlining ethical guidelines, ensuring transparency and accountability throughout the project lifecycle. It is also vital to conduct impact assessments and establish strategies for bias mitigation, which helps organizations maintain ethical standards while leveraging AI technologies. By proactively addressing these areas, businesses can bolster trust and integrity in their AI initiatives, ultimately leading to more successful project outcomes.
Risk and Benefit analysis for AI uses cases
Risk and benefit analysis for AI use cases is a vital step in the feasibility study phase, allowing organizations to balance potential rewards against associated risks. By evaluating factors such as data integrity, compliance with regulations, and the effectiveness of intelligent automation, businesses can make informed decisions on the deployment of AI solutions. This process helps identify areas where AI can significantly enhance operations, ensuring that the adoption of automation aligns with organizational objectives and minimizes unforeseen challenges:
- Assessing potential rewards from intelligent automation.
- Identifying risks related to data privacy and integrity.
- Aligning AI initiatives with organizational goals.
- Evaluating compliance with industry regulations.
Review Data Strategy, Data Quality, Governance readiness for AI
Reviewing the data strategy, data quality, and governance readiness for generative artificial intelligence is a fundamental aspect of the feasibility study for AI projects. Organizations must ensure that their data management frameworks are robust, especially when leveraging cloud computing solutions. A well-defined data strategy aligned with effective governance practices not only enhances machine learning outcomes but also safeguards compliance and fosters trust in AI initiatives.
Identify uses cases based on Impact and Deployment
Identifying use cases based on impact and deployment is critical in the feasibility study of AI projects, particularly in sectors like health care. For instance, integrating a chatbot can significantly enhance customer experience by providing instant support and addressing inquiries efficiently. Data science methods enable organizations to pinpoint areas where AI applications will deliver the most value, ensuring that investments truly resonate with customer needs and amplify operational effectiveness.
AI Solution Design and Roadmap
The AI solution design and roadmap encompass several key aspects essential for successful integration of AI technologies. This includes documenting both external and internal compliance requirements to ensure the safe use of AI, developing models, frameworks, and tools that enhance productivity through analytics, and establishing a comprehensive governance roadmap with timelines and resource requirements. Additionally, a thorough cost analysis for the proposed AI solutions is vital for aligning expectations and maximizing ROI within the ecosystem. AI solution design and roadmap
Documenting External and Internal Compliance Requirements for safe use of AI
Documenting external and internal compliance requirements is critical in the AI solution design and roadmap process. This documentation ensures that organizations address strategic management and risk management needs effectively, safeguarding revenue and enhancing competitive advantage in the marketplace. By aligning workforce training with compliance frameworks, companies can better prepare their teams for the safe use of AI technologies while minimizing potential legal or operational risks:
- Identify relevant external regulations and internal policies.
- Develop a compliance checklist for AI deployment.
- Establish training programs to support workforce understanding of compliance standards.
- Regularly assess compliance readiness to adapt to changing regulations.
Developing AI models, frameworks and tools
Developing AI models, frameworks, and tools involves a systematic approach that integrates research and leadership to establish effective policies for deployment. Organizations need to focus on deep learning techniques to enhance their AI assets, ensuring that models are robust and aligned with specific business objectives. By prioritizing a structured development process, companies can address their operational challenges and maximize the potential benefits of AI integration:
- Establish research foundations to support model development.
- Implement leadership strategies for effective project management.
- Define clear policies that guide AI deployment and ensure compliance.
- Utilize deep learning to enhance the functionality and accuracy of AI tools.
Developing a comprehensive roadmap for governance, timelines, milestones, resource requirements
Developing a comprehensive roadmap for governance, timelines, milestones, and resource requirements is essential for successful AI solution design and implementation. An effective roadmap ensures that organizations have a clear proof of concept for their AI initiatives, enhancing customer service and operational efficiency. By integrating knowledge-sharing practices and defining the necessary infrastructure, businesses can streamline the deployment process, ultimately leading to improved outcomes and timely project completion:
- Establish governance frameworks to oversee AI projects.
- Set clear timelines and milestones for each project phase.
- Identify required resources to support implementation.
- Incorporate proof of concept stages to validate ideas.
- Focus on enhancing customer service through targeted AI solutions.
- Develop strategies for ongoing knowledge transfer within teams.
Develop a comprehensive cost analysis for proposed AI solution
Developing a comprehensive cost analysis for a proposed AI solution is crucial in the AI consulting process. This analysis should encompass factors such as architecture, integration with the internet of things, and the implementation of robotic process automation to provide a clear financial picture. By addressing ethics in AI deployment and considering insights from the Boston Consulting Group, organizations can ensure that investments are not only cost-effective but also aligned with their strategic goals and ethical standards.
Cost Analysis Factors | Description | Importance |
---|---|---|
Architecture | The structural design of the AI solution. | Helps in understanding the technical requirements and costs. |
Internet of Things | Integration with IoT devices for data collection. | Enhances the functionality and data accuracy of AI applications. |
Robotic Process Automation | Automation of repetitive tasks. | Increases efficiency and reduces operational costs. |
Ethics | Consideration of ethical implications of AI use. | Ensures compliance and builds trust with stakeholders. |
Boston Consulting Group Insights | Using expert analysis to guide decisions. | Provides benchmarks and strategic recommendations. |
Pilot Testing and Implementation Support
Pilot testing and implementation support involve guiding organizations through the AI pilot project process, focusing on key areas such as predictive analytics and virtual assistant functionalities. This phase includes collaboration with stakeholders to evaluate and troubleshoot solutions, ensuring that adoption aligns with organizational goals. Post-implementation support further enhances effectiveness by providing assistance in refining the AI landscape.
Guiding organizations through AI pilot project implementation
Guiding organizations through AI pilot project implementation requires a structured approach that prioritizes change management and addresses cultural shifts within the team. By leveraging tools such as Databricks, companies can ensure data sustainability while effectively tracking progress and outcomes. An audit of current processes is essential to identify areas for improvement, ultimately creating a pathway for successful integration of AI technologies into everyday operations:
Key Focus Areas | Details | Benefits |
---|---|---|
Change Management | Strategies to manage transitions effectively. | Improves team adaptation to AI technologies. |
Databricks | Platform for collaborative data management. | Fosters data sustainability. |
Culture | Shifting organizational mindset toward innovation. | Enhances acceptance and support for AI initiatives. |
Audit | Review of current processes and capabilities. | Identifies strengths and areas for improvement. |
Working with stakeholders to evaluate, troubleshoot and refine solution
Involving stakeholders in the evaluation, troubleshooting, and refinement of AI solutions fosters creativity and builds confidence in the outcomes. By leveraging insights from various departments, organizations can effectively address challenges encountered during pilot testing, ensuring that the AI initiatives, particularly those integrating large language models or digital twin technologies, align seamlessly with business objectives. This collaborative approach not only enhances data transformation processes but also prepares teams for successful adoption, paving the way for sustained benefits in their AI journey.
Post implementation support
Post-implementation support plays a vital role in the AI consulting process, particularly as organizations undergo digital transformation. This support ensures that solutions, such as those enhancing the supply chain, are functioning optimally and achieving the desired results. By collaborating with experts, like those at Capgemini, businesses can leverage ongoing assistance to address challenges, refine processes, and streamline pricing strategies, ultimately securing sustained success and maximizing their investment in AI technologies.
Training, Adoption, and Capacity Building
Effective AI consulting encompasses training, adoption, and capacity building to enhance organizational proficiency. This includes developing training materials on tools and best practices, facilitating workshops to build internal capabilities, and establishing a knowledge transfer plan for seamless handover. Such initiatives not only improve user experience but also align with strategic planning to leverage technologies like sentiment analysis, particularly in the manufacturing sector.
Developing training material for staff on tools and best practices
Developing training materials for staff on tools and best practices is essential for maximizing computer science applications in organizations. By equipping employees with the knowledge of predictive analytics and machine learning, companies can enhance their ability to derive business value from AI technologies. These training resources should focus on practical applications, such as how AI can improve decision-making in mergers and acquisitions, making the prediction process more efficient and reliable.
- Identify the required skills for staff in AI initiatives.
- Create comprehensive training resources that address these skills.
- Integrate practical examples aligned with business objectives.
- Facilitate interactive workshops to engage staff and encourage adoption.
Developing workshops to build internal capacity
Developing workshops to build internal capacity is essential for aligning business processes with emerging technologies. By leveraging tools such as Microsoft Azure, these workshops equip teams with necessary skills in software development and statistics, fostering a deep understanding of AI applications. As organizations become more cognizant of the evolving digital landscape, these educational initiatives help cultivate a culture of innovation, ultimately enhancing operational effectiveness and responsiveness to market demands.
Developing a knowledge transfer plan for organizational handover
Developing a knowledge transfer plan for organizational handover is essential in ensuring that employees fully grasp the capabilities and functions of artificial intelligence solutions. This plan should encompass comprehensive training in data analytics and hybrid intelligence, allowing teams to effectively leverage these innovative technologies. By facilitating knowledge sharing and practical application, organizations can empower their staff to enhance productivity and decision-making, ultimately driving a successful AI adoption journey.
Frequently Asked Questions
What steps are involved in developing an AI strategy?
Developing an AI strategy involves defining clear objectives, assessing available data and technology, identifying key stakeholders, and creating an implementation roadmap that aligns with business goals. Regular evaluation and adaptation are essential for success.
How is the feasibility of AI projects assessed?
The feasibility of AI projects is assessed by evaluating technical requirements, cost implications, timeline, data availability, and alignment with business goals. This process ensures project viability and maximizes chances of successful implementation. For more insights into AI project assessment and consulting, explore our AI consulting services.
What is included in AI solution design and roadmap?
AI solution design and roadmap encompass
- needs assessment
- system architecture
- data strategy
- integration planning
- proof of concept
- scalability considerations
- implementation timeline
- post-launch support
for a successful deployment.
How does pilot testing and implementation support work?
Pilot testing and implementation support involve evaluating web applications to ensure functionality and usability. This process includes user feedback, troubleshooting, and continuous improvement, enabling smooth transitions to full deployment while addressing specific business needs effectively. Web applications
What training and capacity building activities are recommended for AI adoption?
Effective training and capacity building for AI adoption should include workshops on AI fundamentals, hands-on project experience, and ongoing support. Tailored learning paths enable teams to integrate AI effectively into their workflows and enhance productivity.
Conclusion
The typical scope of the AI consulting process is crucial for organizations aiming to successfully integrate artificial intelligence into their operations. By identifying relevant use cases and conducting thorough needs assessments, companies can align AI initiatives with their strategic goals, maximizing operational efficiency and value. Implementation support and effective training further ensure that teams are equipped to leverage AI technologies effectively. Ultimately, a robust AI consulting process empowers businesses to navigate the complexities of digital transformation while driving innovation and competitiveness. Digital fractal technologies is a Canadian based AI consulting company with full service offering from consulting, implementation of web and mobile apps and AI solutions, serving Edmonton, Calgary, Vancouver, Houston, Dallas, Atlanta areas. Contact us for a free consultation.
Category
- Artificial Intelligence (7)
- Cyber Security (2)
- Cybersecurity (6)
- Digital Transformation (15)
- Industry News (13)
- Infographics (10)
- Mobile App Development (59)
- Uncategorized (3)
All Tag
Archives
- January 2025
- October 2024
- September 2024
- July 2024
- June 2024
- May 2024
- April 2024
- February 2024
- January 2024
- December 2023
- November 2023
- October 2023
- September 2023
- July 2023
- June 2023
- May 2023
- March 2023
- December 2022
- November 2022
- October 2022
- April 2022
- March 2021
- February 2021
- January 2021
- December 2020
- November 2020
- October 2020
- September 2020
- August 2020
- July 2020
- June 2020